In 2015, Paul-Erik Veel, an associate (now a partner) at the litigation boutique Lenczner Slaght, was preparing a client to take the stand in a medical-malpractice case. “I told him about the kinds of conduct that juries respond well to,” says Veel. The client, who came from the world of peer-reviewed studies and double-blind trials, asked Veel if he had empirical evidence to back up his advice. Veel’s response was blunt: “No, that’s not how the law works.”
Lawyers consider themselves custodians of a bespoke profession, rooted in intuition and experiential knowledge. The tactics that litigators deploy in court — to persuade judges, influence juries and rhetorically outmaneuver opposing counsel — are often the product of conventional wisdom that senior practitioners have passed down through anecdote. When Veel disclosed this fact to his medical client, he was bothered. Tradition has its virtues, but empirical questions frequently arose in his work. Shouldn’t he give empirical answers?
Today, Veel is working hard to inject a bit of science into the art of litigation. At Lenczner Slaght, he has overseen the creation of the Supreme Court of Canada Leave Project, a machine-learning tool that can determine the likelihood of a given case to be granted leave at the nation’s top court.
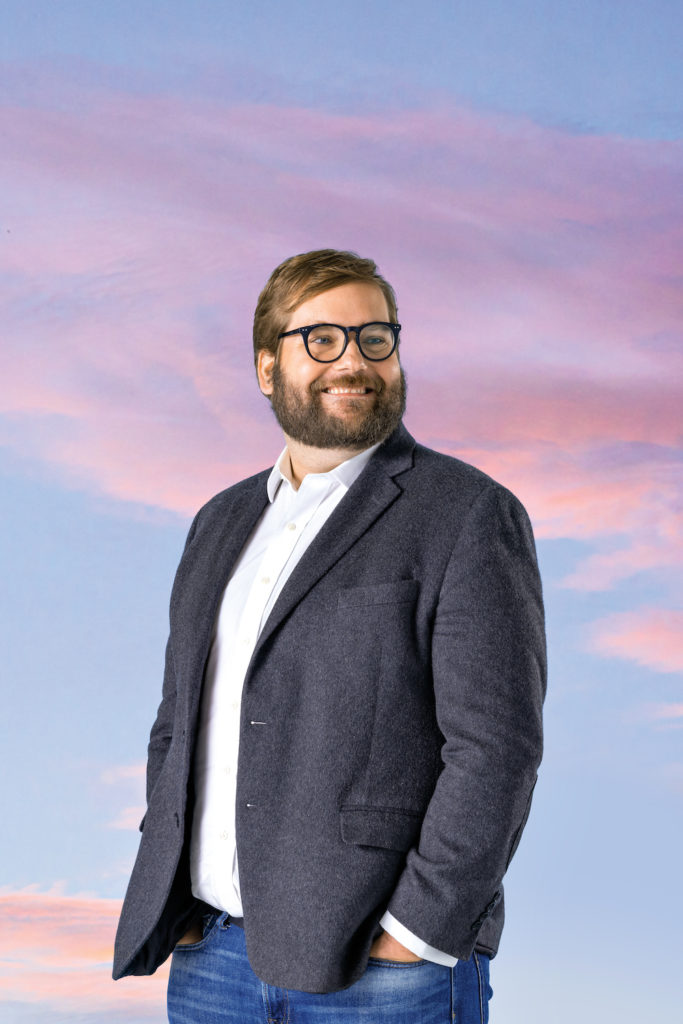
Paul-Erik Veel
Lenczner Slaght
As a starting point, the probability is always pretty low. Over the past decade, the court has reviewed an average of 532 leave applications a year; in most years, it has accepted less than 10 percent of them. Still, some long shots are longer than others. When clients are contemplating the time and money necessary to apply for leave at the Supreme Court, they typically want to know whether their odds are decent or straight-up terrible. By launching the SCC Leave Project, Veel is able to answer that question with more authority than ever before.
To create the tool, Veel and his co-workers first built a database of every leave application filed at the court since January 2018, just after Richard Wagner took over as chief justice. Katie Glowach, an associate at the firm with a background in computer information systems, designed a data-input portal, making it as straightforward as possible. Using drop-down menus that she created, Veel and an intern sorted each application into a broad range of categories, including the type of case, whether leave was granted, whether the case generated dissents in lower courts and whether the client was an individual, a company or a government.
Once the data entry was complete, Veel designed the machine-learning algorithm with an open-source application he found online. The program could then review a new leave application and, based on its knowledge of what the court has accepted in the past, determine its chance of success.
Each week, between Monday (when the Supreme Court discloses which leave decisions it will announce) and Thursday (when it actually makes the announcements), Veel runs the pending cases through the algorithm. The program then assigns a probability of success to each case. Finally, Veel sorts them into four categories: “long shots,” which have less than a 1-percent chance of success; “unlikely contenders,” which sit in the 1-to-5-percent range; “possible contenders,” where the odds are between 5 and 25 percent; and “cases to watch,” where the probability of getting leave exceeds 25 percent. These predictions are then published on the Lenczner Slaght blog in advance of the final announcement. This allows readers to see for themselves whether the algorithm is doing its job.
So far, the team has a lot to be proud of. Among the first 123 applications that the SCC Leave Project has analyzed, 50 percent of the “cases to watch” received leave, compared with 17 percent of the “possible contenders,” 3 percent of the “unlikely contenders” and none of the “long shots.” “That gave us confidence,” says Veel. “On average, our model was getting things right.”
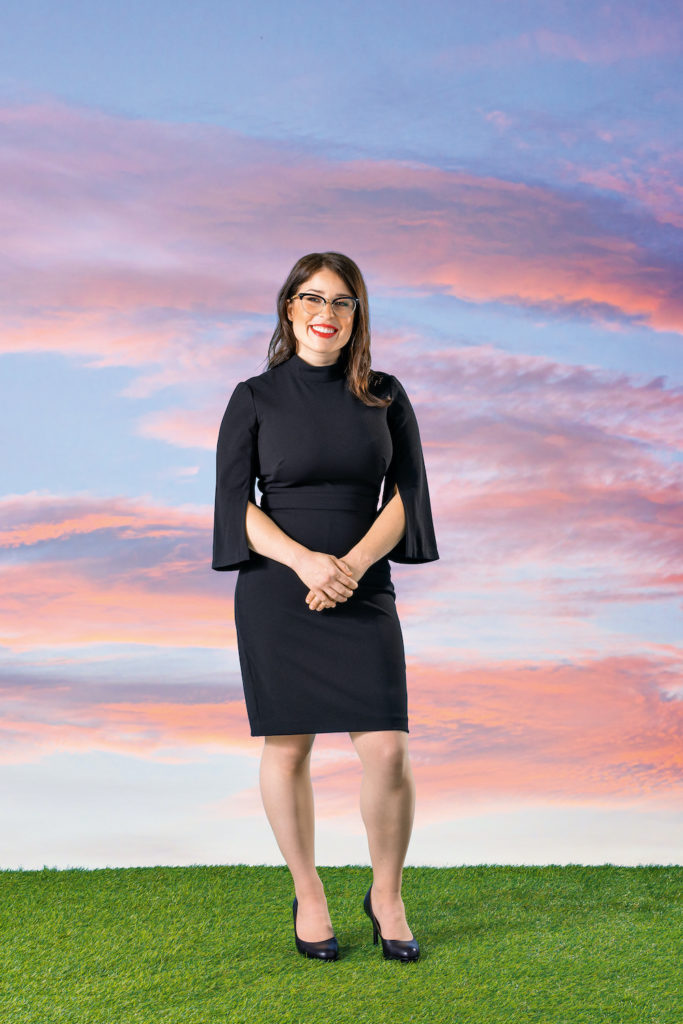
Katie Glowach
Lenczner Slaght
Veel has also been able to fact-check the conventional wisdom of his field. Historically, litigators have assumed that the nation’s top court favours criminal and constitutional cases, as well as applications from the federal government. Armed with data, Veel put those theories to the test. As it turns out, government applications do have a competitive advantage. (As an elected institution, Veel explains, it seems to enjoy priority status.) The court does not, however, have a bias toward criminal and constitutional files. (According to Veel, such cases are slightly overrepresented at the Supreme Court, but that’s not because leave applications in these areas have an intrinsic upper hand. The real reason, he points out, is that criminal and constitutional matters often arrive at the court through alternative judicial pathways like an automatic appeal or a request by the federal government for an opinion.)
The goal of the SCC Leave Project isn’t just to wow people with its predictive capabilities. The idea is also to serve clients. Any litigant who retains Lenczner Slaght can now get a data-based estimate as to how strong a leave application might be. “If the model goes to the one-, two- or three-percent range — as it often does — I can confidently say to the client, ‘It’s not worth it,’” says Veel. “If we’re into the 15-, 20- or 25-percent range, I can say, ‘This is a case the Supreme Court will look at hard.’”
Ultimately, the client must decide what to do based on gut instinct and tolerance for risk. But that’s okay. “We’re not seeking to replace legal experience and intuition,” says Glowach, “but rather to supplement it.”
Monique Jilesen, a partner at the firm who has supported the team in this move toward a more empirical approach to advocacy, concurs. “As lawyers, we’re in the judgment business,” she says. “Experience matters deeply. But experience supported by data is more powerful still.”
This story is from our Winter 2021 Issue.
Photography by Kyle Jeffers, hair and makeup by Alanna Chelmick.